Have you ever wondered what plants you might see around you, or on your next walk? This service could help you answer that question.
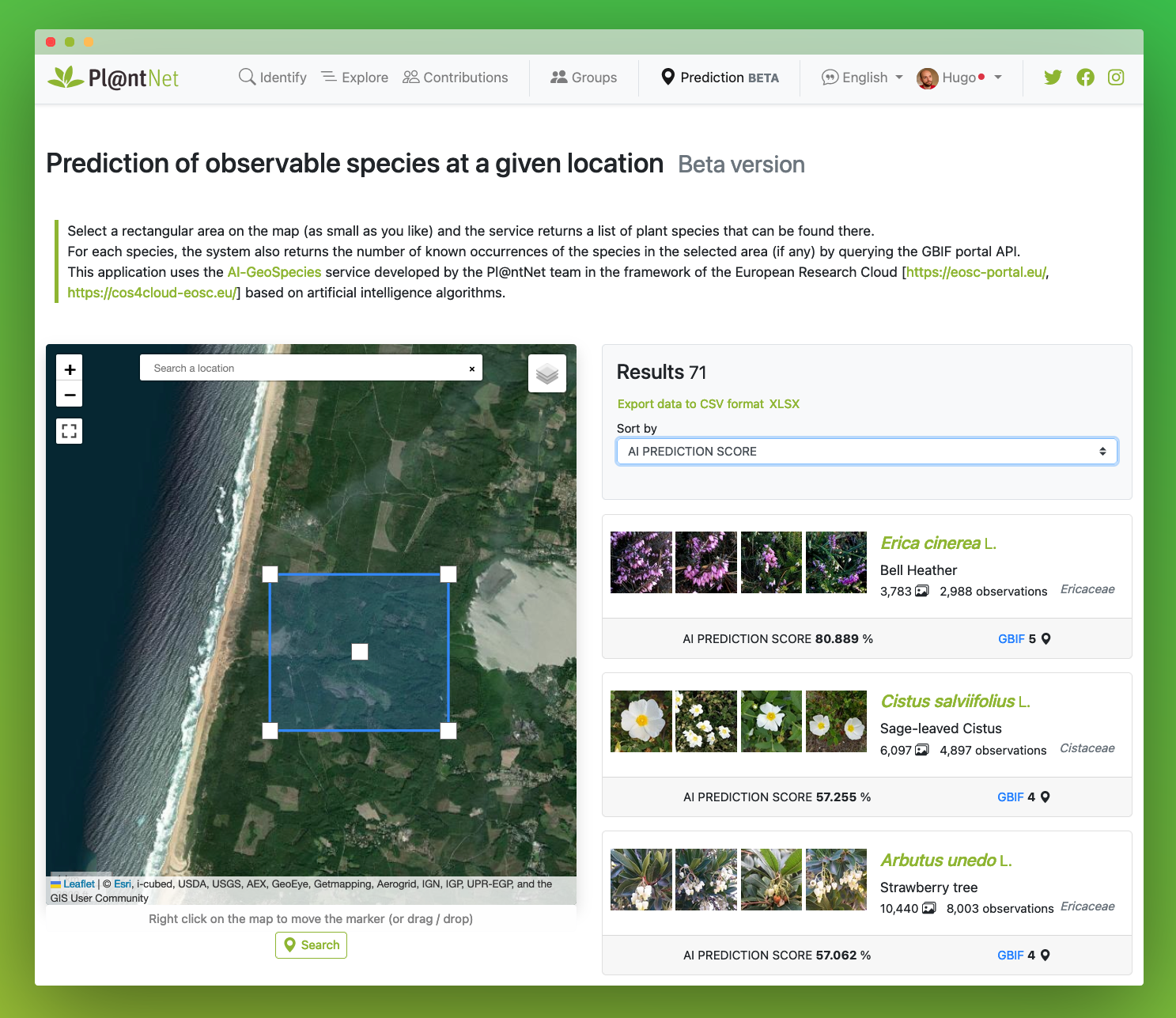
Consulting the plant species potentially present in a given place can be of great interest to both environmental management professionals and novices in botany.
Such a service can, for example, improve the planning of observation campaigns, or facilitate the management of certain areas by anticipating the appearance of new species. It can also be a very useful tool to feed the discussion between citizens and decision-makers on the subject of land use planning and the impact of human infrastructures on biodiversity.
This original service, called GeoPl@ntNet, enables you to find out which plant species have already been observed or are potentially present in this area by selecting a point on a map and drawing a rectangle around it. It is based on Pl@ntNet data, of course, but also more generally on data from GBIF, the largest biodiversity data platform in the world to which Pl@ntNet contributes (see the article on this subject). In order to predict species in places where there is little or no data, the application uses an artificial intelligence algorithm (called a Deep-SDM*), trained to predict the species present from high-resolution satellite data (from the IGN) and environmental data (temperature, precipitation, soil type, etc.). This technology is the result of several thesis works carried out within the Pl@ntNet research team [1,2,3] and has been implemented within the framework of the European project Cos4Cloud, which focuses more generally on new technologies for citizen science. The service is currently mainly adapted for predictions in metropolitan France. You can use it outside France, but in this case, only known occurrences are exploited and you will not benefit from AI predictions in areas where there is no data.
Do not hesitate to let us know your perception and your suggestions for improvement.
The Pl@ntNet team.
*Deep-SDM: Potential geographic distribution model of species based on the training of a convolutional deep neural network.
1. Botella, C., Joly, A., Bonnet, P., Monestiez, P., & Munoz, F. (2018). A deep learning approach to species distribution modelling. In Multimedia Tools and Applications for Environmental & Biodiversity Informatics (pp. 169-199). Springer, Cham.
2. Deneu, B., Servajean, M., Bonnet, P., Botella, C., Munoz, F. and Joly, A., 2021. Convolutional neural networks improve species distribution modelling by capturing the spatial structure of the environment. PLoS computational biology, 17(4), p.e1008856. https://doi.org/10.1371/journal.pcbi.1008856
3. Deneu, B., Joly, A., Bonnet, P., Servajean, M. and Munoz, F., 2022. Very High Resolution Species Distribution Modeling Based on Remote Sensing Imagery: How to Capture Fine-Grained and Large-Scale Vegetation Ecology With Convolutional Neural Networks?. Frontiers in Plant Science, 13. https://doi.org/10.3389%2Ffpls.2022.839279